[ad_1]
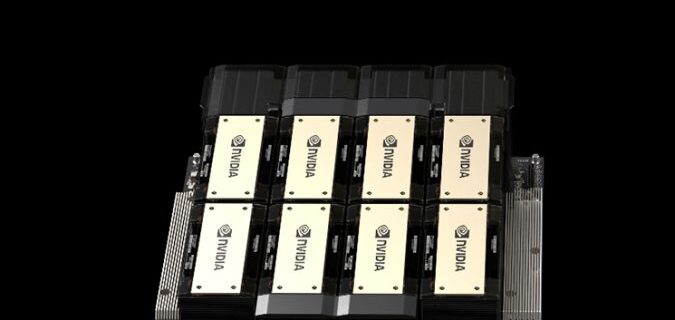
There were no startling surprises in the latest MLPerf Inference benchmark (4.0) results released yesterday. Two new workloads — Llama 2 and Stable Diffusion XL — were added to the benchmark suite as MLPerf continues to keep pace with fast-moving ML technology. Nvidia showcased H100 and H200 results, Qualcomm’s Cloud AI 100 Ultra (preview category) and Intel/Habana’s Gaudi 2 showed gains. Intel had the only CPU-as-accelerator in the mix.
Juniper Networks was a first-time participant showcasing the importance of the network. There was a combined Red Hat-Supermicro submission leveraging OpenShift AI. Cloud infrastructure provider Wiwynn was another new submitter.
Overall, the number of submitters has been fairly stable in recent years. There were 23 this time including ASUSTeK, Azure, Broadcom, Cisco, CTuning, Dell, Fujitsu, Giga Computing, Google, Hewlett Packard Enterprise, Intel, Intel Habana Labs, Juniper Networks, Krai, Lenovo, NVIDIA, Oracle, Qualcomm Technologies, Inc., Quanta Cloud Technology, Red Hat, Supermicro, SiMa, and Wiwynn. MLPerf Inference v4.0 included over 8500 performance results and 900 Power results from 23 submitting organizations.
Missing were the heavy chest-thumping bar charts from Nvidia versus competitors as the rough pecking order of inference accelerators, at least for now, seems to be settled. One of the more interesting notes came from David Salvator, Nvidia director of accelerated computing products, who said inference revenue was now 40% of Nvidia’s datacenter revenue.
“Inference has become an important part of our data center activities and business,” said Salvator, “On our last earnings call, we stated that it’s about 40% of our of our data center revenue last year. Part of the reason [is] we’re seeing this crossover where inference is becoming the more dominant workload. [The reason] is that once an application deploys, those applications are generally running 24/7. With training, you complete a training run, and you’re basically you’re done, at least for a while. With inference, once that app is deployed, it’s running all the time, and starts to consume sort of a larger portion of the overall AI workload.”
Since its launch in 2018, MLPerf has steadily become a fixture in the accelerator market, and perhaps a little less exciting in the process but useful for comparing diversely-configured systems for specific use cases. There is no single winner, a la Top500. David Kanter, executive director of MLCommons, the organizer of MLPerf benchmark efforts, has consistently noted that to get value from the results it’s necessary to dig into the data and compare systems on an apples-to-apples basis.
“To me, the point of benchmarking is to align the whole industry together. This is helping to inform buyers, helping them make decisions and understand how systems, whether they’re on premise systems, cloud systems, embedded systems, perform on relevant workloads. So that if you’re looking to buy a system to run a large language model inference, you can use benchmarks to help guide you. At the same time for folks in the industry, who are producing the solutions, these benchmarks help us understand how to optimize how to improve,” said Kanter.
“We’ve added two new benchmarks here. They’re both generative AI benchmarks. The first one is Stable Diffusion XL, which is text to image generation. We [also] added the Llama 2 large language model for question and answering. Now, both of these require that we come up with latencies for the server, server mode, and actually also the offline mode, as well. One of the things that I wanted to highlight here is this chart of what the parameter count looks like for MLPerf for inference models over time. You can see that sort of just like AI in general, it’s very much a hockey stick up into the right. I think in our first round of MLPerf Inference (v.5) the largest model was like 200 million parameters and now we’re at 70 billion,” he said.
(The first MLPerf inference results were released in 2019 with five benchmarks focused on three tasks, image classification, object detection, and machine translation. (see HPCwire coverage, MLPerf Expands Toolset; Launches Inferencing Suite) roughly a year after the first training results)
MLCommons provided a deeper look into its decision-making process in adding the two new benchmarks which is posted on the MLCommons site. The composition of the team doing that work — Thomas Atta-fosu, Intel (Task Force Chair); Akhil Arunkumar, D-Matrix; Anton Lokhomotov, Krai; Ashwin Nanjappa, NVIDIA; Itay Hubara, Intel Habana Labs; Michal Szutenberg, Intel Habana Labs; Miro Hodak, AMD; Mitchelle Rasquinha, Google; Zhihan Jiang, Nvidia — reinforces the idea of cooperation among rival companies.
Practically speaking, digging out value from the results requires some work. With this round, MLPerf results are being presented on a different platform — Tableau — and, at least for me, there’a learning curve to effectively use the powerful platform. That said the data is there. Per past practice, MLCommons invites benchmark participants to submit statements about their entries and those are included at the end of the article. Be warned: sifting the marketing chaff from the insight wheat can be challenging.
Nvidia Keeps Rolling
Nvidia remains king of the broad AI accelerator mountain and the lone submitter to tackle all workloads.
MLCommons typically holds an overview pre-briefing for media and analysts, in which participants can comment on results but refrain from making direct competitive comparisons. Individual companies are free to have separate briefings to score competitive points.
Never lacking competitive zeal, Nvidia was relatively modest during its private briefing prompting one analyst to ask, “One thing seems to be missing. You normally show a slide that has a bar chart for all the different benchmarks that you submitted, how they compare to previous [runs] and or how they compare to competition. I didn’t see that in this in this slide deck. What’s up with that?”
Salvator said, “Well, so the results will be widely available on the ML Commons website, we decided for this round to focus on the newer workloads with a particular focus on the Llama 2 workload, [which] we think really represents the current state of the art. We did submit on every workload, and the numbers are there. In the case of the Ilama 2 we did show one competitive submission from Intel/Habana with Gaudi2…the main other accelerator who submitted. There were some other CPU-centric results that were submitted that, frankly, we are quite a bit faster than those.”
Instead Salvator focused on the growing complexity of inferencing, advances made in Nvidia’s TensorRT-LLM compiler, and its effectiveness in boosting H100 and H200 performance Llama 2. The H200-based systems were entered in the preview category because at the time of submission they were’t yet available but now they are, he said, citing Lenovo as one vendor with a H200 system now available.
Much of the material covered by Salvator had been touched on during its GTC24 conference a week ago. He discussed Nvidia MGX, its modular reference design for using different chassis and thermal footprints, and Nvidia Inference Microservices (NIMs).
Asked about forthcoming Blackwell GPUs, B100 and B200, and their drop-in compatibility with existing H100 and H200 systems, Salvator said, “We have not designed B200 to be drop-in compatible with an H200 CTS system. The drop-in compatible side is focused more on the B100, because we have a pretty significant installed base of H100 base servers and a lot of our partners know how to build those servers. So that ability to easily swap in a B100 base board gets them to market that much faster. B200 will require a different chassis design. It’s not going to be drop-in compatible with H200 systems.”
Intel/Habana Tout Performance and Cost
In recent years Intel has edged into the MLPerf arena touting its Gaudi accelerator line as a high-performance, lower cost alternative to Nvidia GPUs and its 5th-gen Xeon CPUs as very well suited for systems in which inference or training is a smaller portion of a mixed workload.
In making comparisons based on the latest MLPerf Inferencing run, Intel presented both performance and a cost comparison for Gaudi 2 versus Nvidia H100 on the Stable Diffusion XL and Llama 2 workloads.
Here’s a portion of Intel’s vendor statement:
“The Intel Gaudi 2 accelerator, a 7nm processor, presented solid performance results for state-of-the-art models on MLPerf Inference. On Stable Diffusion XL, Gaudi 2 accelerators delivered 6.26 and 6.25 for offline samples-per-second and server queries-per-second, respectively, and, for Llama v2-70B, 8035.0 and 6287.5, offline and server tokens-per-second, respectively. Given the strong customer demand for Hugging Face TGI (text generation interface), Intel submitted Llama results using the TGI serving toolkit which supports continuous batching and tensor parallelism, thus enhancing the efficiency of real-world LLM scaling. The Intel Gaudi software suite continues to increase coverage of our highest customer demand LLM and multi-modal models.”
At Intel’s separate pre-briefing, Itay Hubara, principal engineer & senior researcher, Habana Labs, said, “We’re very excited to make the submission of TGIK which is an open source serving hugging face which is the most [used] the the serving social with the highest demand. You can see it normalized by price performance on the right side based on a third party assessment. I feel like it’s a fair comparison.”
He said the cost comparison was based on system cost, not operation costs, but didn’t provide more detail.
Ronak Shah, AI product director, Xeon, Intel, added, “Gaudi has been taking leaps and bounds to get to that point where we deliver that ease-of-use, whether through the standard frameworks like pytorch, or leveraging Hugging Faces TGI in this submission, which enables you to be able to get that out of box performance and deliver these types of results with a standard framework that is available in the industry.”
This was the first appearance of Intel 5th-Gen Xeon in MLPerf and Shah presented its results versus 4th-Gen Xeon.
“What you can see here (slide above) is that compared to 4th-Gen Xeon and the prior submission, we increased our performance by a factor of 1.42x. What excites me is that we launched 4th-Gen Xeon at the beginning of 2023 and launched 5th-Gen at the end of 2023, and within one year, it was a combination of hardware and enhancements from 4th-Gen to 5th Gen, along with software optimizations that was able to deliver this 1.42x increase in performance. [The] exciting thing is the timeline at which we were able to deliver such a large gain, and that we were able to really leverage some underlying architecture enhancements,” said Shah.
Shah noted Intel had five partners submitting this time around. “The fact that we have five partners that submitted is indicative of the fact that they also are recognizing that this is where Xeons key strengths are; it’s in the area of when you have mixed general purpose workloads or a general purpose application and you’re infusing AI into it.” The five partners were Cisco, Dell, Quanta, Supermicro, and WiWynn.
Overall, the range of systems submitted in MLPerf was fairly wide. Bulleted here are excerpts from a few of the vendor submitted statements (full list of statements appended below):
Juniper Networks: “For MLPerf Inference 4.0, Juniper Networks submitted a suite of tests for a Llama 2, 70 billion-parameter large language model (LLM) running over a Juniper validated design (JVD) consisting of a spine-leaf network topology with a rail-optimized design. The multi-node data center setup was powered by a Juniper AI-optimized Ethernet fabric, including QFX Series switching with ROCEv2 for inter-GPU communication. Testing and validation were performed in the Juniper AI lab on NVIDIA A100 and H100 clusters, featuring intra-rail and inter-rail combinations. This is the first ever multi-node Ethernet submission to MLCommons.”
Qualcomm: “For the v4.0 round, Qualcomm has introduced the AI inference accelerator Cloud AI 100 Ultra and submitted it for ‘Closed Preview’ mode evaluation. The early preview results for Cloud AI 100 Ultra demonstrate its exceptional performance at low power, as evidenced by its performance across ML Benchmarks. Compared to the Cloud AI 100 Pro submissions, all Cloud AI 100 Ultra submissions exhibit a 2.5 to 3 times performance improvement while consuming less than 150W power per accelerator. In addition to NLP and Computer Vision networks, we have introduced the GenAI Stable Diffusion XL submission. Our partners Dell, HPE, and Lenovo have also submitted their Preview results for Cloud AI 100 Ultra cards.”
Red Hat & Supermicro: “Supermicro, builder of Large-Scale AI Data Center Infrastructure, and Red Hat Inc, the world’s leading provider of enterprise open source solutions, collaborated on the first ever Red Hat OpenShift AI MLPerf Inference v4.0 submission. This submission demonstrates the flexibility of OpenShift AI’s model serving stack to support open source LLM runtimes such as vLLM by using the custom runtime feature. We are also proud that we are the only result in this round to submit results on both GPT-J-6b and llama-2-70b using vLLM on Nvidia GPUs without any quantization or model compilation.”
Wewynn: “In the edge category, we benchmarked the ES200G2 equipped with two NVIDIA L40S GPUs for edge applications such as image recognition or other AI applications. In the data center category, we benchmarked the ES200G2 with an Intel 5th Gen Xeon processor, which can be formed into a server pool to perform various tasks. Both results show that the platform is capable of running popular AI frameworks and achieving good performance.”
Next up is the MLPerf Training expected in the June time frame.
Link to MLCommons press release, https://www.hpcwire.com/off-the-wire/new-mlperf-inference-benchmark-results-highlight-the-rapid-growth-of-generative-ai-models/
Link to MLPerf Inference 4.0 datacenter results, https://www.hpcwire.com/off-the-wire/new-mlperf-inference-benchmark-results-highlight-the-rapid-growth-of-generative-ai-models/
MLPERF VENDOR SUBMITTED STATEMENTS (lightly edited)
Asus
In our journey towards excellence in the MLPerf v4.0 Inference Benchmark, ASUSTek efforts transcend mere optimization of performance and reliability to advance AI technologies deployed in all fields. We have embraced the ethos of community engagement, recognizing its pivotal role in fostering collaboration, knowledge sharing, and collective advancement in the field of machine learning.
Performance Tuning:
Together with ASUS ESC8000-E11P, Intel 4th Gen Xeon Scalable Processor and NVIDIA H100 PCIe GPU solutions, at the heart of our efforts lies a relentless pursuit of performance optimization. Leveraging the immense computational power of GPU servers, we’ve embarked on a quest to fine-tune every facet of our infrastructure. Through meticulous experimentation and innovative techniques, we’ve achieved remarkable enhancements in throughput, latency, and efficiency. Our solutions are meticulously crafted to harness the full potential of GPUs, delivering unmatched speed and responsiveness in real-world inference scenarios.
Reliability Enhancements:
On top of MLperf Inference v4.0, we’ve placed a premium on fortifying the reliability of our GPU servers. Recognizing the critical importance of stability in mission-critical applications, we’ve implemented robust mechanisms to mitigate downtime, prevent bottlenecks, and enhance fault tolerance. Rigorous testing and validation procedures ensure that our infrastructure consistently delivers dependable performance under diverse workloads and operating conditions.
Community Engagement:
Beyond technical prowess, our commitment to the MLPerf community stands as a cornerstone of our success. We actively participate in knowledge exchange forums, contribute insights, and collaborate with fellow practitioners to drive innovation forward. By sharing best practices, lessons learned, and insights gained from our journey, we contribute to the collective wisdom of the community, fostering an environment of collaboration and mutual growth.
Conclusion:
In the realm of the MLPerf 4.0 Inference Benchmark, our achievements are not merely measured in terms of performance metrics and reliability benchmarks. They are equally rooted in our dedication to community engagement and collaborative spirit. As we continue to push the boundaries of what’s achievable, our commitment to driving collective advancement in the field of machine learning remains unwavering. Together, we stride towards a future where innovation knows no bounds, fueled by the power of collaboration and shared knowledge.
Broadcom
As a leader in virtualization technologies, VMware by Broadcom has empowered global enterprises by providing innovative infrastructure solutions for data center management which can help customers build, run, and manage applications efficiently, securely and flexibly. For machine learning (ML) and artificial intelligence (AI) workloads, our software solutions work with most hardware vendors to enable these workloads at scale. Broadcom, Dell and NVIDIA have collaborated to bring the magic of virtualization to accelerator data centers for MLPerf Inference v4.0. In addition to legacy benchmarks, Broadcom, Dell and NVIDIA have submitted stellar results for the new Stable Diffusion (text-to-images) benchmark. Our results deliver near bare metal or better performance with the added virtualization benefits of data center management.
We ran MLPerf Inference workloads on Dell XE9680 with 8 x virtualized NVIDIA SXM H100 80GB GPUs and Dell R760 with 2x virtualized NVIDIA L40S 80GB GPUs with vSphere 8.02 and NVIDIA vGPU. The virtual machines used in our tests were allocated with only 32 out of 120 – 224 available CPUs and 128 GB out of 1T – 1.5T available memory. We used just a fraction of the system capacity. Hence, customers can use the remaining CPU & memory capacity on the same systems to run other workloads, save costs of ML/AI infrastructure and leverage the virtualization benefits of VMware vSphere for managing data centers. The comparison of our results with bare metal presented above show that vSphere 8.0.2 with NVIDIA virtualized GPUs is the goldilocks zone for AIML workloads.
Cisco
Enterprises across all industries are recognizing the true potential of AI/ML. Artificial intelligence (AI) and machine learning (ML) are opening up new ways for enterprises to solve complex problems.
Cisco is a new member of the MLCommons community. Cisco successfully submitted results for MLperf v4.0 Inference in partnership with Intel for datacenter category in Large Language Model (LLM), Image classification (Vision), Object detection(Vision) and Speech-to-text (Speech).
Cisco submitted the Inference results for Cisco UCS C240 M7 server with Intel Xeon 5th generation processors. The Cisco UCS C240 M7 server with Intel 5th generation Xeon scalable processors delivers leading performance and improved efficiency in a 2RU form factor, the ideal platform for AI inferencing.
As a new member of MLCommons community, Cisco continues to support the community’s efforts in benchmarking server solutions for various AI training, inference & HPC workloads. In the latest MLPerf 4.0 Inference, Cisco submitted results for the Intel Xeon 5th Gen processor on Cisco UCS C240 M7 platform, and the results showing that system has achieved excellent performance in most of the inference models.
CTuning
In this submission round, we have tested the 2nd generation of the MLCommons CM-MLPerf workflow and CK playground to automatically benchmark diverse edge servers, laptops and cloud providers including AWS and Cirrascale on Nvidia, Intel, Amazon and Qualcomm-based commodity hardware (8683 performance results out of 9528 and 905 power results out of 988).
The goal of the CM-MLPerf is to provide a single and human-friendly command line, a simple GUI and extensible Python, C++ and network implementation templates to run all MLPerf inference benchmarks from different vendors and submit results in a unified and automated way.
The new version of CM-MLPerf was developed by the cTuning foundation and cKnowledge following the request from MLCommons after the last submission round and thanks to the feedback from the MLCommons members and the research community (ACM/IEEE MICRO’23 and SuperComputing’23).
For the first time, CM-MLPerf workflow managed to automate all the edge+datacenter workloads (llama2 submission done using llama2-7b model) as well as diverse implementations from Nvidia, Intel, Qualcomm, Neural Magic and MLCommons.
We are also very proud to benchmark Qualcomm Cloud AI 100 systems in the cloud for the first time using MLCommons CM and thank Qualcomm for their support. We also thank colleagues from Intel, Nvidia and Google for their feedback and suggestions.
We invite everyone to use and enhance MLCommons CM-MLPerf automation and participate in a new project to automatically co-design high-performance and cost-effective AI applications and systems using MLPerf and CM as a collaborative engineering effort.
The following graph, produced by the CM-LPerf explorer plugin, shows latencies of the edge models on our submission systems (both using Nvidia RTX 4090) to be among the best latencies submitted to MLPerf inference. Nvidia RTX 4090 also shows impressive offline and server performance as can be seen in our datacenter results.
Dell Technologies
Dell stands out with the broadest range of GenAI solutions, covering everything from desktops to data centers to the cloud. The company is at the forefront of the AI evolution, with the Dell PowerEdge XE server series laying the foundation for this transformative journey.
In the realm of MLPerf inferencing v4.0 benchmark testing, Dell Technologies showcases its commitment by submitting 281 results across various models, including tests with the new Llama2-70b, Stable Diffusion XL, GPT-J, utilizing CPUs and accelerators from Qualcomm, Broadcom, NVIDIA, and Intel. The testing covers a wide array of products, demonstrating Dell’s capability to cater to diverse AI workloads with their PowerEdge server family.
The Dell PowerEdge XE series, particularly with NVIDIA’s Tensor Core H100 GPUs, demonstrates exceptional performance in areas like large language models, image classification, and more. Additionally, Dell’s efforts in system efficiency are highlighted by the PowerEdge XR5610 with NVIDIA L4 GPU, optimizing performance for Edge workloads.
The Dell PowerEdge XE accelerated server family continues to deliver tremendous performance gains across several benchmarks. Here are some of the latest highlights:
The PowerEdge XE9680 with 8 NVIDIA H100 Tensor Core GPUs continues to deliver Dell’s best performance results in large language models, text-to-image, speech-to-text, language processing, image classification, and recommendation.
Stellar results for the 4 GPU Direct Liquid Cooled Dell PowerEdge XE9640 and air-cooled PowerEdge XE8640 in GenAI models, image classification, object detection, speech-to-text, language processing, summarization, medical image segmentation and more.
Excellent system performance per watt per GPU with the Dell PowerEdge XR5610 and NVIDIA L4 GPU for Edge workloads
Dell invites customers to explore these advancements through test drives at their worldwide Customer Solution Centers, offering collaboration with their Innovation Lab and access to Centers of Excellence for deeper insights into AI solutions.
Fujitsu
Fujitsu offers a fantastic blend of systems, solutions, and expertise to guarantee maximum productivity, efficiency, and flexibility delivering confidence and reliability. Since 2020, we have been actively participating in and submitting to inference and training rounds for both data center and edge divisions.
In this round, we submitted entries to the datacenter closed division using two systems. The first system is PRIMERGY CDI, equipped with 16xL40S installed in an external PCIe BOX. The second system is GX2560M7, equipped with 4xH100-SXM inside the server. We also submitted entries to datacenter closed power division using PRIMERGY CDI.
PRIMERGY CDI can be used as a single node by installing up to 20 GPUs in three external PCI-BOXes. Additionally, system configuration can be adjusted according to the size of training and inference workloads. In this round, we installed 16xL40S on the PRIMERGY CDI system and ran stable diffusion and gptj. Measurement results are displayed in the figure below. We confirmed the performance, as shown in the figure, using the system equipped with multiple L40S.
Our purpose is to make the world more sustainable by building trust in society through innovation. With a rich heritage of driving innovation and expertise, we are dedicated to contributing to the growth of society and our valued customers. Therefore, we will continue to meet the demands of our customers and strive to provide attractive server systems through the activities of MLCommons.
Giga Computing
Giga Computing Technology, a subsidiary wholly owned by GIGABYTE, is the enterprise unit split off from GIGABYTE that designs, manufactures, and sells servers, server motherboards, immersion solutions, and workstations.
As one of the founding members of MLCommons, Giga Computing has continued to support the community’s efforts in benchmarking server solutions for various AI training & inference workloads. Following the last v3.1 Inference benchmarks, Giga Computing submitted a powerful GIGABYTE G593-SD1 system configured with the latest 5th Gen Intel Xeon Scalable Processors and eight NVIDIA H100 SXM5 GPUs in the latest round of MLPerf Inference v4.0. The system features high data bandwidths and meticulously optimized data processing configurations. And the results speak for themselves, demonstrating great efficiency while maintaining top-of-the-line performance across all benchmarked tasks. Our exceptional results in the latest benchmarks underscore our commitment to delivering top-tier capabilities and optimization.
Our focus at Giga Computing is on continual improvement, exemplified by our provision of remote testing and public benchmarks for system evaluations. We are dedicated to driving efficiency and pioneering advanced cooling technologies, such as immersion and DLC, to address the forthcoming surge in power consumption. Stay tuned as we continue to push the boundaries of computing excellence with Giga Computing.
Google Cloud
NVIDIA GPUs combined with Google Cloud’s infrastructure technologies provide industry-leading scale and performance. In August, we announced that A3 VMs are now generally available; powered by NVIDIA 8 H100 Tensor Core GPUs in a single VM, A3s are purpose-built to train and serve demanding gen AI workloads and LLMs. A3 is able to reach supercomputing capabilities by reaching 26 exaflops of AI performance.
For the MLPerf Inference v4.0 benchmark testing, Google submitted 20 results, including the new Stable Diffusion XL and Llama 2 (70B) results, using A3 VMs. The Stable Diffusion XL and Llama 2 results were within 1-4% of the peak performance demonstrated by NVIDIA’s submissions. The strong A3 VM results are a testament to Google Cloud’s close partnership with NVIDIA to build workload-optimized end-to-end solutions specifically for LLMs and gen AI.
HPE
Hewlett Packard Enterprise (HPE) successfully submitted results in partnership with NVIDIA, Qualcomm, and KRAI, demonstrating a range of high-performing inference systems for the datacenter on computer vision (CV), natural language processing (NLP), generative AI (GenAI), and large language models (LLMs). HPE server performance results were included in Datacenter-Closed, Datacenter-Open, and Datacenter-Network divisions.
HPE submitted AI Inference results on these systems:
- HPE Cray Supercomputing (SC) XD670 (with 8x NVIDIA H100 SXM 80GB, 700W TDP*)
- HPE ProLiant DL380a Gen11 server (with 4x NVIDIA H100 PCIe 80GB, 400W TDP*)
- HPE ProLiant DL380a Gen11 server (with 4x NVIDIA L40S PCIe 48GB, 300W TDP*)
- HPE ProLiant DL380a Gen11 server (with 8x Qualcomm Cloud AI 100 Ultra 128GB, 150W TDP*)
Highlights include:
- The HPE Cray SC XD670 with NVIDIA H100 SXM demonstrated the highest performing result for NLP with Bert 99.0 Offline scenario
- The HPE ProLiant DL380a with 4x NVIDIA H100 PCIe demonstrated the highest performing result on the Llama2 70B model for four or less PCIe attached GPUs.
- The HPE ProLiant DL380a with 4x NVIDIA L40S demonstrated good performance in its class of GPUs for CV, NLP, GenAI and LLMs.
- HPE submitted its first MLPerf Inference preview result on CV and NLP with 8x Qualcomm Cloud AI 100 Ultra accelerators in an HPE ProLiant DL380a Gen11 server.
Many thanks to KRAI’s collaboration achieving high-performance and energy efficiency for Qualcomm Cloud AI 100 Ultra accelerators.
* TDP per GPU or accelerator
Intel
Intel submitted MLPerf Inference v4.0 results for Intel Gaudi 2 AI processors, and, for the first time, 5th Gen Xeon Scalable processors. The results show Intel’s commitment to delivering the full spectrum of AI products to address wide-ranging customer AI requirements.
The Intel Gaudi 2 accelerator, a 7nm processor, presented solid performance results for state-of-the-art models on MLPerf Inference. On Stable Diffusion XL, Gaudi 2 accelerators delivered 6.26 and 6.25 for offline samples-per-second and server queries-per-second, respectively, and, for LLama v2-70B, 8035.0 and 6287.5, offline and server tokens-per-second, respectively. Given the strong customer demand for Hugging Face TGI (text generation interface), Intel submitted LLama results using the TGI serving toolkit which supports continuous batching and tensor parallelism, thus enhancing the efficiency of real-world LLM scaling. The Intel Gaudi software suite continues to increase coverage of our highest customer demand LLM and multi-modal models.
Intel remains the only CPU vendor to submit MLPerf results. Intel has submitted MLPerf results for four generations of Xeon products, starting in 2020. Intel’s submissions for 5th Gen Intel Xeon Scalable processors with Intel Advanced Matrix Extensions (AMX) demonstrate that CPUs have great performance for general-purpose AI workloads. Intel’s 5th Gen Xeon results improved by a geomean of 1.42X compared to 4th Gen Xeon’s results in MLPerf Inference v3.1 last year due to hardware and software improvements.
For GPT-J with software optimizations – including continuous batching – Intel’s Xeon submission showed ~1.8X performance gains compared to v3.1 submission. Similarly, DLRMv2 showed ~1.8X performance gains and 99.9 accuracy due to MergedEmbeddingBag and other optimizations utilizing AMX.
Intel is proud of its collaboration with OEM partners – Cisco, Dell, Quanta, Supermicro, and WiWynn – to deliver their own MLPerf submissions.
With ongoing software updates and optimizations, Intel expects continued advances in performance and productivity for its accelerators and CPUs.
Juniper Networks
For MLPerf Inference 4.0, Juniper Networks submitted a suite of tests for a Llama 2, 70 billion-parameter large language model (LLM) running over a Juniper validated design (JVD) consisting of a spine-leaf network topology with a rail-optimized design. The multi-node data center setup was powered by a Juniper AI-optimized Ethernet fabric, including QFX Series switching with ROCEv2 for inter-GPU communication. Testing and validation were performed in the Juniper AI lab on NVIDIA A100 and H100 clusters, featuring intra-rail and inter-rail combinations. This is the first ever multi-node Ethernet submission to MLCommons.
Juniper is thrilled to collaborate with MLCommons to accelerate artificial intelligence (AI) innovation and make AI data center infrastructure simpler, faster, and more economical for companies around the world to deploy. Generative AI such as LLama has pushed the performance boundaries of compute, storage, and networking systems. Training these models is a massive, parallel processing problem that is dependent on robust networking solutions. AI workloads have unique characteristics and present new requirements for the network, but solving tough challenges such as these is what Juniper has been doing for over 25 years. For AI cluster infrastructure to move from the early adopter stage to mass market, we must use open technologies to harness the collective power and innovation of the industry ecosystem.
Juniper is committed to an operations-first approach to help our customers manage the entire AI data center network lifecycle with market-leading capabilities in intent-based networking, AIOps and 800Gb Ethernet. Open technologies such as Ethernet and our Apstra data center fabric automation software eliminate vendor lock-in, take advantage of the industry ecosystem to push down costs and drive innovation, and enable common network operations across AI training, inference, storage, and management networks. In addition, rigorously pre-tested, validated designs, such as those Juniper has submitted to MLCommons, are critical to ensure that customers can deploy secure data center infrastructure on their own.
KRAI
Established in 2020 in “Silicon Fen” (Cambridge, UK), KRAI are the purveyors of premium benchmarking and optimization solutions tailored for engineering ultra-efficient and cost-effective Computer Systems for Artificial Intelligence. The KRAI team has participated in all nine MLPerf Inference rounds, a feat achieved by only three other submitters out of 60+ since 2019.
The v4.0 round marked 3 years of the exceptionally strong collaboration between Qualcomm and KRAI. To celebrate the occasion, we focused on enabling outstanding results with Cloud AI 100 Ultra accelerators being previewed in this round. In particular, a GIGABYTE G293-Z43 2U server equipped with 16 single-wide Ultra accelerators delivered over 900,000 samples/second on ResNet50 and nearly 15,500 samples/second on RetinaNet. With 64 AI cores per Ultra accelerator, this achievement represents linearly scaling to 1,024 AI cores in a single system, with the previous highest achievement being 288 cores (with 18 Pro accelerators). The magic sauce/source for ultra performant, efficient and scalable inference has been shared with the community as a new public release of the acclaimed KRAI Inference Library Technology (KILT) codebase. In this round, KILT was used in submissions from Qualcomm, KRAI, HPE, Dell, Lenovo and CTuning.
As another proud moment for KRAI, we collaborated with Google on reproducing and optimizing LLM submissions using the latest generation Tensor Processing Units. Google Cloud customers are welcome to reproduce the TPU-v5e results using workflows automated with the KRAI X technology.
We thank HPE for providing access to a ProLiant DL385 server equipped with 8 Cloud AI 100 Standard accelerators and 200GbE networking gear, which enabled the only Network Closed submission in this round. Crucially, the networking upgrade from 10GbE in the previous round allowed us to scale the more bandwidth-hungry RetinaNet benchmark, in addition to the bandwidth-light BERT benchmark.
Lenovo
Lenovo is dedicated to delivering smarter technology solutions for all, including hardware, software, and more. To achieve this, we conduct research and testing using MLPerf Inference v.4.0, allowing us to showcase our leading results in benchmarking.
Through our partnership with MLCommons, Lenovo has been able to demonstrate these results quarterly through the MLPerf benchmarking. Our collaboration with NVIDIA and Intel on important AI tasks such as Image Classification, Medical Image Segmentation, Speech-to-text, and Natural Language Processing has enabled us to achieve leading results.
We are proud to have competed on these tasks using our ThinkSystem SE360 with 2x NVIDIA L4 and the SE450 and SE455 with 2x NVIDIA L40 Edge Servers. These collaborations have allowed us to consistently improve our technology for our customers based on our leading benchmarks.
Our partnership with MLCommons provides valuable insights into how we compare against the competition, sets customer expectations, and allows us to continuously enhance our products. Through this collaboration, we can work closely with industry experts to create growth and ultimately deliver better products for our customers, who are our top priority.
NVIDIA
We are excited to demonstrate the incredible inference performance of the NVIDIA accelerated computing platform in MLPerf Inference v4.0. The NVIDIA HGX H100 platform integrates up to eight H100 Tensor Core GPUs with high-speed interconnects and delivered nearly 3x more performance on the GPT-J test compared to the prior round thanks to our TensorRT-LLM software. This inference optimizer and runtime improves ease of use and extensibility through an open-source modular Python API for defining, optimizing, and executing new architectures and enhancements as LLMs evolve.
We were also thrilled to make our debut submission using the NVIDIA HGX H200 AI supercomputing platform – powered by the latest H200 Tensor Core GPUs. HGX H200, using a high-performance custom thermal solution, delivered up to 45% more performance than the HGX H100 on the new Llama 2 70B LLM test. And, the NVIDIA GH200 Grace Hopper Superchip – which combines the NVIDIA Grace CPU with the NVIDIA Hopper GPU in a versatile, easy-to-deploy module – extends the great performance of H100 GPUs across LLMs, text-to-image generative AI, and recommenders.
The NVIDIA AI platform delivers innovation across the full technology stack, accelerates the entire AI workflow end-to-end – from data preparation to model training to deployed inference from cloud to edge – and achieves great performance across a broad range of AI models. It’s also available from every major cloud and server maker, and offers the quickest path to production AI and enterprise-grade support with NVIDIA AI Enterprise.
We are thrilled to see 14 NVIDIA partners, including ASUSTek, Azure, Broadcom, Cisco, Dell, Fujitsu, GigaComputing, Google, HPE, Lenovo, Oracle, Quanta Cloud Technology, Supermicro, and Wiwynn submit great inference results, with both on-prem and cloud solutions spanning the breadth of the NVIDIA data center GPU portfolio.
We also wish to commend the ongoing work MLCommons is doing to bring benchmarking best practices to computing, enabling peer-reviewed apples-to-apples comparisons of AI and HPC platforms to better understand and compare product performance across diverse workloads.
Oracle
Oracle Cloud Infrastructure (OCI) offers AI Infrastructure, Generative AI, AI Services, ML Services, and AI in our Fusion Applications. Our AI infrastructure portfolio includes bare metal instances and virtual machines powered by NVIDIA H100, NVIDIA A100, and NVIDIA A10 GPUs.
The inference benchmark results for the high-end BM.GPU.H100.8 instance demonstrate that OCI provides high performance that at least matches that of other deployments for both on-premises and cloud infrastructure. These instances provide eight NVIDIA GPUs per node. In addition to inferencing, for training workloads each node can be clustered using a high performance RDMA network to tens of thousands of GPUs.
OCI’s BM.GPU.H100.8 instance provides the highest available performance with NVIDIA GPUs on OCI, as of March 2024.
Quanta Cloud Technology
Quanta Cloud Technology (QCT), a global datacenter solution provider enabling diverse HPC and AI workloads, has been named among the MLPerf inference list in the latest MLPerf results released by MLCommons.
QCT participated in the latest round of MLPerf Inference v4.0 and submitted results to the data center closed division, including new models of stable diffusion and llama2 for different system configurations.
One showcased configuration featured QCT’s cutting-edge platform, the newly available QuantaGrid S74G-2U with NVIDIA Grace Hopper Superchip. The coherent memory between the CPU and GPU with NVLink C2C interconnect can improve memory-intensive AI inference. QCT achieved outstanding performance in multiple AI tasks in the data center categories.
The QuantaGrid D54U-3U is an acceleration server designed for AI/HPC. Supporting two 5th Gen Intel Xeon Scalable processors, this 3U system features support for four dual-width accelerator cards or up to eight single-width accelerator cards, providing a comprehensive and flexible architecture optimized for various AI/HPC applications. This time, QCT validated the results with four NVIDIA H100 PCIe and four NVIDIA L40S PCIe cards, respectively.
Another configuration showcased QCT’s QuantaGrid D54X-1U with Intel Xeon Scalable Processors in CPU-only inference scenarios. QCT’s server with a CPU-only configuration was validated for its capability to perform excellently in general-purpose AI workloads with Intel AMX instruction sets.
Moving forward, QCT remains committed to delivering comprehensive hardware systems, solutions, and services to both academic and industrial users. The company will continue to share its MLPerf results with the MLCommons community, contributing to the advancement of MLPerf inference and training benchmarks.
Qualcomm Technologies, Inc.
The Qualcomm Cloud AI inference accelerators leverage the company’s expertise in advanced signal processing and power efficiency to deliver high throughput, low power AI inference processing in both Datacenter and Edge environments.
For the v4.0 round, Qualcomm has introduced the AI inference accelerator Cloud AI 100 Ultra and submitted it for “Closed Preview” mode evaluation. The early preview results for Cloud AI 100 Ultra demonstrate its exceptional performance at low power, as evidenced by its performance across ML Benchmarks. Compared to the Cloud AI 100 Pro submissions, all Cloud AI 100 Ultra submissions exhibit a 2.5 to 3 times performance improvement while consuming less than 150W power per accelerator. In addition to NLP and Computer Vision networks, we have introduced the GenAI Stable Diffusion XL submission. Our partners Dell, HPE, and Lenovo have also submitted their Preview results for Cloud AI 100 Ultra cards.
In a first for Cloud AI 100, CTuning has submitted results using a Amazon EC2 DL2q cloud instance powered by 8x Cloud AI 100 Standard accelerators, achieving performance equivalent to a standalone server. CTuning has also submitted MLPerf benchmarks using the Cirrascale Quad AI 100 Cloud instance, powered by 4x Cloud AI 100 Pro Accelerators, achieving results comparable to standalone systems.
Qualcomm’s MLPerf Inference v4.0 results have surpassed its own previous records in terms of peak offline performance and power efficiency across all categories. The 2U datacenter server platform, equipped with 16x Qualcomm Cloud AI 100 Ultra accelerators (150W TDP), has achieved an impressive throughput of over 902K ResNet50 inf/Sec in Preview mode. It has also set new all-time-high power efficiency, achieving 275 QPS/Watt for ResNet50, 5.2 QPS/Watt for RetinaNet, and 10.2 QPS/Watt for BERT.
These results from Qualcomm’s submissions are made possible by using KRAI’s X and KILT technologies. Qualcomm and Snapdragon are trademarks or registered trademarks of Qualcomm Incorporated. Qualcomm Cloud AI and Snapdragon are products of Qualcomm Technologies, Inc. and/or its subsidiaries.
Red Hat + Supermicro
Supermicro, builder of Large-Scale AI Data Center Infrastructure, and Red Hat Inc, the world’s leading provider of enterprise open source solutions, collaborated on the first ever Red Hat OpenShift AI MLPerf Inference v4.0 submission. Red Hat OpenShift AI is a flexible, scalable MLOps platform with tools to build, deploy, and manage AI-enabled applications.
GPU A+ Server, the AS-4125GS-TNRT has flexible GPU support and configuration options: with active & passive GPUs, and dual-root or single-root configurations for up to 10 double-width, full-length GPUs. Furthermore, the dual-root configuration features directly attached 8 GPUs without PLX switches to achieve the lowest latency possible and improve performance, which is hugely beneficial for demanding scenarios our customers face with AI and HPC workloads.
Red Hat OpenShift makes creating, scheduling and monitoring your AI/ML workloads easier and more secure. OpenShift Operators discover, configure and monitor your GPUs, storage devices, and network devices, providing ease of use, flexibility and security.
Red Hat OpenShift AI is a flexible, scalable MLOps platform with tools to build, deploy, and manage AI-enabled applications. Built using open source technologies, it provides trusted, operationally consistent capabilities for teams to experiment, serve models, and deliver innovative apps. Red Hat OpenShift AI (previously called Red Hat OpenShift Data Science) supports the full lifecycle of AI/ML experiments and models, on-premise and in the public cloud.
This submission demonstrates the flexibility of OpenShift AI’s model serving stack to support open source LLM runtimes such as vLLM by using the custom runtime feature. We are also proud that we are the only result in this round to submit results on both GPT-J-6b and llama-2-70b using vLLM on Nvidia GPUs without any quantization or model compilation.
SiMa
SiMa.ai leads the way in edge AI technology, setting a new standard in performance and energy efficiency. We are delighted to share our results in this latest MLPerf benchmarking report, where we surpassed the FPS by 7% to 16% from August 2023 submission across all the categories.
In the edge AI sector, where limited power and demanding tasks constantly create tension between power and efficiency, we are able to make great strides in FPS while still improving the FPS/W across all the workloads from our previous MLPerf 3.1 submission. This metric is a critical indicator of how many frames our system can process per watt of electricity consumed.
Our FPS increase, especially in the SingleStream mode of more than 16%, is one of the most impressive results in the MLPerf v4.0 submission, since SingleStream of batch 1 performance is a predominant workload in real applications. This has been possible due to significant enhancements in the MLA Runtime platform software, in optimizing the end-to-end model execution. The real power of our advancements lies in translating these improvements beyond the benchmarks to real-world benefits for our customers. They experience significantly enhanced performance of all models unlocking a new level of value across a wide range of edge AI applications.
SiMa.ai’s participation and performance in MLPerf is part of a broader growth strategy where we are paving the way for faster, more powerful solutions today, and in the next generations to come. We’re not just making a technical upgrade; this is a strategic leap forward that solidifies our leadership in edge AI performance, efficiency, and innovation.
SuperMicro
Supermicro excels in AI infrastructure solutions, showcasing remarkable performance in the MLPerf Inference v4.0 competition with submissions in both closed and open divisions within the data center inference category.
Supermicro’s mission is to deliver application-optimized systems for a spectrum of workloads. One standout example is the SYS-821GE-TNHR, a customizable solution designed for the NVIDIA HGX H100 8-GPU platform. This system, tailored through our building block approach, caters to customers’ specific requirements and workload needs. Moreover, we now offer liquid cooling options for the latest NVIDIA HGX-based systems, as well as PCIe-based systems, enabling deployments to leverage higher TDP CPUs and GPUs without thermal throttling.
Our GPU servers are meticulously crafted to handle large datasets and high-demand workloads efficiently. They offer expedited access to storage, reduced latency, and improved storage bandwidth, translating into enhanced productivity and faster task completion. Leveraging NVIDIA GPUs and advanced access methods like local DMA and RDMA, alongside high-performance networking through multiple NICs and switches, Supermicro GPU servers excel in AI, machine learning, and HPC tasks.
SYS-521GE-TNRT server, equipped with the L40S GPU, supports up to 10 PCIe-based GPGPUs via a PCIe 5.0 dual-root switch, delivering exceptional processing power. With 48GB of GDDR6 memory and a theoretical performance of 91.6 TFLOP, the L40S GPU is optimized for AI media and graphics applications, making it invaluable for high-performance computing tasks. Additionally, featuring dual 4th Generation Intel Xeon Scalable Processors, up to 8TB of memory capacity, and ample storage options with 24 hot-swap NVMe/SATA/SAS drive bays, this configuration offers scalability and efficiency for intensive computing tasks.
Supermicro offers a diverse range of GPU systems for any environment, consistently delivering high performance in multiple MLPerf benchmarks. Moving forward, we remain committed to fine-tuning our systems to provide optimized experiences and performance for our customers.
Wiwynn
Wiwynn is a leading cloud IT infrastructure provider for hyperscale data centers. Our main areas of interest include advancements in Cloud, AI, 5G, and edge computing. Exceptionally, we produce high-quality servers for a wide range of applications, including artificial intelligence.
In the latest round of MLPerf Inference v4.0 testing, Wiwynn submitted ES200G2 benchmark results in two categories: edge and data center. The Wiwynn ES200G2 is a 2U server tailored to meet the various demands of telecom usage, including edge applications, inference hosts for 5G service management, and data centers.
In the edge category, we benchmarked the ES200G2 equipped with two NVIDIA L40S GPUs for edge applications such as image recognition or other AI applications. In the data center category, we benchmarked the ES200G2 with an Intel 5th Gen Xeon processor, which can be formed into a server pool to perform various tasks. Both results show that the platform is capable of running popular AI frameworks and achieving good performance.
Wiwynn’s corporate mission is to “Provide the Best TCO, Workload and Energy Optimized IT Solutions from Edge to Cloud”. Wiwynn will continue to work towards this goal and participate in community activities. Our commitment to innovation and excellence is reflected in our participation in industry benchmarks such as MLPerf Inference v4.0, where we strive to demonstrate the capabilities of our products and contribute to the advancement of the field.
Related
[ad_2]
Source link